Transform Your Business with Enterprise AI Solutions | VisionBot
Innovative Enterprise AI Solutions for Every Industry
Transforming Industries with Tailored AI Solutions
Real-World Applications
- Manufacturing: Incorporate predictive maintenance, quality control and supply chain optimisation into production lines.
- Healthcare: Use AI technologies to improve diagnostic instruments, treatment plans and healthcare systems for better patient care.
- Finance: Transform the financial sector and use AI algorithms for risk assessment, fraud detection as well as personalized banking services.
Why VisionBot's Enterprise AI?
- Cutting-Edge Technology: Be ahead in innovation with our cutting-edge AI technologies.
- Expert Team: Collaborate with an AI team focused on knowing and fulfilling the needs of your business.
- Scalability and Flexibility: Our AI solutions will increase with time and hence they ll remain efficient as long you are doing business.
Elevate Your Business with VisionBot's Enterprise AI
VisionBot’s AI solutions provide a pathway to not just adapt but excel. Our built-in AI tools are engineered to revolutionize your operations, offering efficiency, insights, and agility essential for any successful business. Embrace VisionBot’s AI solutions and confidently face the future, discovering the keys to success.
Order a free demo now and witness the transformative power of enterprise AI with VisionBot’s solutions. Join the ranks of visionary businesses and let us guide you in unleashing the full potential of AI within your organization. VisionBot is shaping a future that’s not only bright but intelligently driven.
Optimization of Maintenance Schedule
ROI Example
- Reduce maintenance costs by 20.5%
- Reduce unplanned downtime by 30% to 50%
- Extend the lifetime of aging assets by 20%
- Achieve 27.5% up to 50% in energy savings
Data Needed
- Historical and Real-time Sensor Data per Machine. Examples: Temperature, Pressure, Vibration, Rotational Speeds, Current, Chemical Properties.
- Historical failure incidents and maintenance work orders.

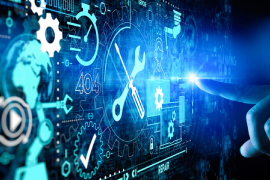
Automated Visual Quality Inspections
ROI Example
- Annotation of Labelled Defect Images (Ideally Tens of Thousands).
- Model Training on annotated Datasets.
Data Needed
- Historical and Real-time Sensor Data per Machine. Examples: Temperature, Pressure, Vibration, Rotational Speeds, Current, Chemical Properties.
- Historical failure incidents and maintenance work orders.
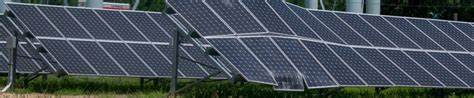
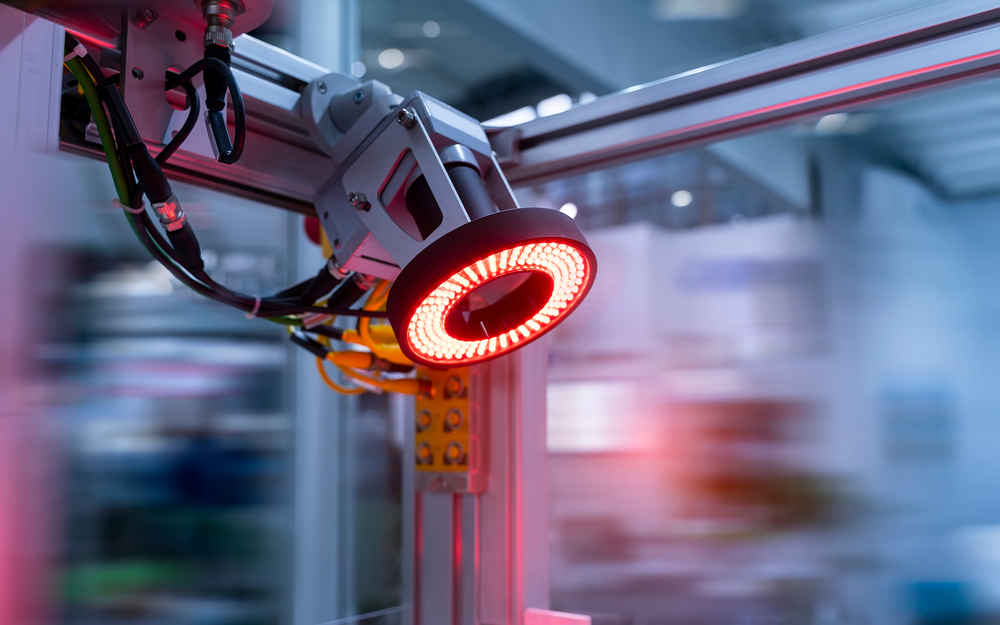
Optimization of Production Plan Schedule
It can analyse Production Scenarios using Sales Forecasts / Pipelines and create valid and optimal plans/schedules that maximizes yield while improving other important production outcomes.
Optimize Product Transitioning/Sequencing (switching from manufacturing product A to product B on the same line), cycle length, while minimizing the costs of transitions and maximizing product availability for customers (taking demand into consideration) – all whilst minimizing inventory on hand.ROI Example
- 10-20% improvement in OTIF planning and scheduling performance
- 25% improvement in Ontime Delivery & customer satisfaction
- Reduced Delta between Sales and Production
- 15% reduction in rolling inventory levels
Data Needed
- Historical Production Yield data
- Historical Production Schedules used
- Order Booked
- Forecast
- Inventory schedules
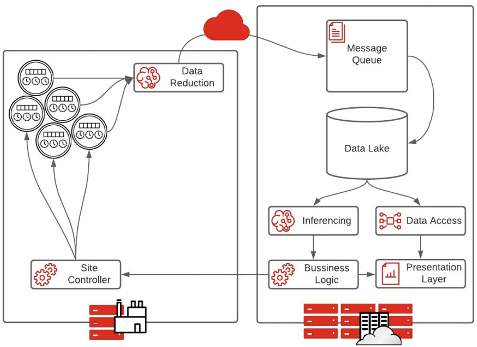
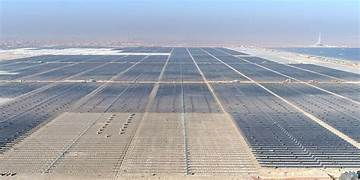
Optimization of Production Process
It can analyse inputs from diverse sensor inputs (Temp, Pressure, Boiler, Humidity, Clean Room etc) and create valid and parameters to increase Production rate, maximizes yield while reducing costs.
A Trained model can detect complex correlation between these parameters and production rate. Further an generate optimal configuration to improve overall equipment effectiveness.ROI Example
- 25 – 30 % production yield improvement.
- 5-10 % reduction in production costs.
- 5- 15 % improvement in Equipment uptime.
Data Needed
- Historic Production Data per Product: yield, parameter values per each production step, total time taken.
- Historic Sensor data: Boiler Load , voltage, pressure, temperature.
- Historic Maintenance data per Machine: work orders, failure.
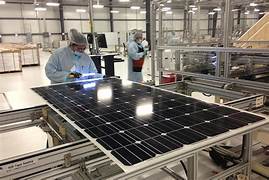
Predictive Maintenance
Predicting supply chain machine failure before it happens to avoid downtime and reduce maintenance costs (e.g. trucks, vessels, airplanes). AI can also recommend optimal time for intervention and best actions to avoid failures.
Business Impact: reduce down time for vehicle and machines, improve safety, decrease spent time on unplanned maintenance, increase customer and driver satisfaction.ROI Example
- Reduce Vehicle Downtime by 15%
- Reduce related delays by 10-15%.
- Reduce Idling times in Service Centres by 15-18%.
Data Needed
- Historical Vehicle Telemetry and Real-time Sensor data (e.g. vibration, voltage, pressure, temperature)
- Historical failures, dates of repairs (work orders), current status for machines and cargo carriers.
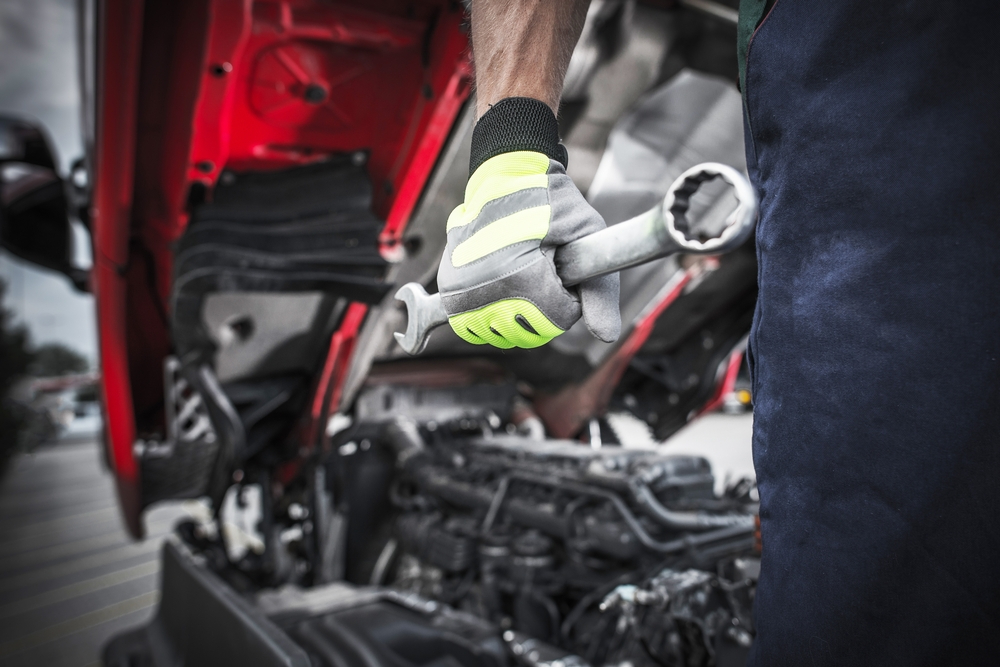

Demand Optimization
Business Impact
ROI Example
- 20 to 30% Reduction in Forecasting Errors of Supply Chain Networks
- Up to 35% Reduction in Lost Sales due to inventory out-of-stock situations
- 10 to 40% Reduction in Warehousing Costs
Data Needed
- Historical Product Sales per stock point
- Product Attributes (e.g. price, category)
- Historical Promotions & Marketing Activities (optional)
- Supply Chain Assets data: trucks, routes , drivers, schedules
- Other data sets (optional): Seasonal, competition offers etc
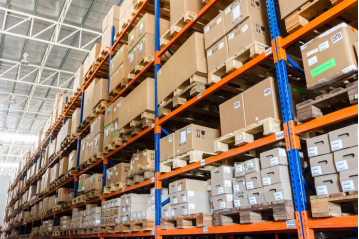
Design Optimization
Business Impact
ROI Example
- An auto company could create 150 new design ideas for a seat bracket. 40% lighter and 20% stronger than the original part
- A design house was able to create a new design that reduced the battery case weight by 40%
Data Needed
- Designs for Products or Parts of interest
- Images for Products and Parts.
